2014 №02 (05) | 2014 №02 (07) |
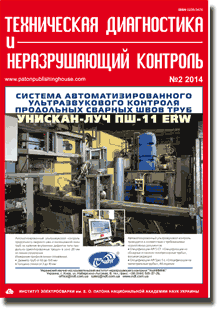
Техническая диагностика и неразрушающий контроль, №2, 2014 стр. 33-36
ИССЛЕДОВАНИЕ МЕТОДОВ СЕГМЕНТАЦИИ РАДИОГРАФИЧЕСКИХ СНИМКОВ СВАРНЫХ СОЕДИНЕНИЙ
В. В. Ремнева, И. Ф. Погребняк, А. В. Шарко
Реферат:
Исследована эффективность методов сегментации радиографических изображений сварных соединений, основанных на вычислении оценки сходимости результатов и заключений экспертов. Определены перспективы использования алгоритма нечеткой кластеризации системы автоматического анализа изображений при радиографическом контроле сварных соединений. На основе результатов экспериментальных исследований показано, что наиболее приемлемым методом сегментации радиографических изображений сварного шва является метод нечеткой кластеризации C?средних. Полученные результаты могут быть использованы при создании программного обеспечения для автоматизированного анализа результатов неразрушающего контроля сварных швов. Это позволит повысить качество контроля сварных соединений и сократить временные затраты на его проведение. Библиогр. 19, рис. 1, табл. 1.
Ключевые слова: радиография, сегментация, изображение, критерии, качество, оценки
Effectiveness of the methods of segmentation of welded joint radiographic images based on calculation of assessment of convergence of results and expert evaluations was studied. Prospects for application of the algorithm of fuzzy clustering of the system of automatic analysis of images at radiographic inspection of welded joints were determined. Proceeding from the results of experimental investigations, it is shown that the method of fuzzy clustering of C-averages is the most acceptable method of segmentation of radiographic images of the weld. Obtained results can be used in development of software for automated analysis of weld NDT results. This will allow improvement of the quality of weld NDT and reducing the time consumption for its performance. Ref. 19, Figures 1, Tables 1.
Keywords: radiography, segmentation, image, criterion, quality, evaluations
1. ГОСТ 7512–82. Контроль неразрушающий. Соединения сварные. Радиографический метод. – М.: Изд-во стандартов, 1982. – 19 с.
2. Alaknanda, Anand R. S., Kumar P. Flaw detection in radiographic weldment images using morphological watershed segmentation technique // NDT & E Int. – 2009. – 42(1). – P. 2–8.
3. Image thresholding for weld defect extraction in industrial radiographic testing / N. Nacereddine, L. Hamami, M. Tridi, N. Oucief // Intern. J. of Information and Communication Eng. – 2007. – 3, № 4. – P. 257–265.
4. Исследование алгоритмов сегментации рентгенотелевизионных изображений сварных швов для автоматического обнаружения дефектов / Я. П. Лазоренко, Е. В. Шаповалов, Т. Г. Скуба и др. // Техн. диагностика и неразруш. контроль. – 2009. – № 4. – С. 37–42.
5. A density-based fuzzy clustering technique for non-destructive detection of defects in materials / P. R. Reza Hasanzadeh, A. H. Rezaie, S. H. H. Sadeghi et al. // NDT&E Int. – 2007. – Vol. 40. – Р. 337–346.
6. Wafaa Al-Hameed, Mayali Y., Picton P. Segmentation of radiographic images of weld defect // J. of Global Research in Computer Science. – 2013. – 4, №7. – P. 1–4.
7. Faramarzi F., Motamedi M. Image processing for radiographic films of weld inspection // Proceedinds of the Intern. Conf. on Image Processing, Computer Vision, and Pattern Recognition. – 2011. – 1. – P. 337–343.
8. Non-parametric histogram-based thresholding methods for weld defect detection in radiography / N. Nacereddine, L. Hamami, M. Tridi, N. Oucief. // Intern. J. of Electrical, Electronic Sci. and Eng. – 2007. – 1, № 9. – P. 159–163.
9. Vaithiyanathan V., Raj A., Venkatraman B. PCA and clustering based weld flaw detection from radiographic weld images // Intern. J. of Eng. and Technology. – 2013. – 5, №3. – P. 2878–2883.
10. Chaudhuri D., Agrawal A. Split-and-merge procedure for image segmentation using bimodality detection approach // Defence Sci. J. – 2010. – 60, № 3. – P. 290–301.
11. Pushpa R. Suri, Mahak. Image segmentation with modified K-means clustering method // Intern. J. of Recent Technology and Eng. (IJRTE). – 2012. – 1, № 2. – P. 176–180.
12. D. Comaniciu, P. Meer. Mean Shift: A Robust Approach Toward Feature Space Analysis // IEEE Transactions on Pattern Analysis and Machine Intelligence. – 2002. – 24, № 5. – P. 3–619.
13. Zhang Y. J. Advances in image and video segmentation. – IBM Press, 2006. – 473 p.
14. Rand W. Objective criteria for the evaluation of clustering methods // J. of the American Statistical Association. – № 6(336). – P. 846–850.
15. Unnikrishnan R., Pantofaru C., Hebert M. Toward objective evaluation of image segmentation algorithms // IEEE Transactions On Pattern Analysis And Machine Intelligence. – 2007. – 29, № 6 – P. 929–944.
16. Rosenberg A., Hirschberg J. V-measure: A conditional entropy-based external cluster evaluation measure // Proc. of the 2007 Joint Conf. on Empirical Methods in Natural Languages Processing and Computational Natural Language Learning. – Prague, June 2007. – P. 410–420.
17. Yet another survey on image segmentation: Region and boundary information integration / J. Freixenet, X. Munoz, D. Raba et al. // ECCV. – 2002. – P. 408–422.
18. Большев Л. Н., Смирнов Н. В. Таблицы математической статистики. – М: Наука, 1983. – 416 c.
19. Кобзарь А. И. Прикладная математическая статистика. – М: Физматлит, 2006. – 816 c.
Поступила в редакцию15.03.2014
Подписано к печати 25.04.2014